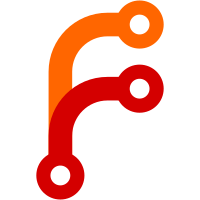
- New AU recognition models trained on extra datasets - Bosphorus, UNBC, FERA2011 - Cleaner and clearer separation of static and dynamic AU models - AU training code cleaned up and instructions added - bug fixes with median feature computation - AU prediction correction (smoothing and shifting) with post processing
382 lines
No EOL
15 KiB
C++
382 lines
No EOL
15 KiB
C++
///////////////////////////////////////////////////////////////////////////////
|
||
// Copyright (C) 2016, Carnegie Mellon University and University of Cambridge,
|
||
// all rights reserved.
|
||
//
|
||
// THIS SOFTWARE IS PROVIDED “AS IS” FOR ACADEMIC USE ONLY AND ANY EXPRESS
|
||
// OR IMPLIED WARRANTIES WARRANTIES, INCLUDING, BUT NOT LIMITED TO,
|
||
// THE IMPLIED WARRANTIES OF MERCHANTABILITY AND FITNESS FOR A PARTICULAR
|
||
// PURPOSE ARE DISCLAIMED. IN NO EVENT SHALL THE COPYRIGHT HOLDERS OR CONTRIBUTORS
|
||
// BE LIABLE FOR ANY DIRECT, INDIRECT, INCIDENTAL, SPECIAL, EXEMPLARY.
|
||
// OR CONSEQUENTIAL DAMAGES (INCLUDING, BUT NOT LIMITED TO, PROCUREMENT OF
|
||
// SUBSTITUTE GOODS OR SERVICES; LOSS OF USE, DATA, OR PROFITS; OR BUSINESS INTERRUPTION)
|
||
// HOWEVER CAUSED AND ON ANY THEORY OF LIABILITY, WHETHER IN CONTRACT,
|
||
// STRICT LIABILITY, OR TORT (INCLUDING NEGLIGENCE OR OTHERWISE) ARISING IN
|
||
// ANY WAY OUT OF THE USE OF THIS SOFTWARE, EVEN IF ADVISED OF THE
|
||
// POSSIBILITY OF SUCH DAMAGE.
|
||
//
|
||
// Notwithstanding the license granted herein, Licensee acknowledges that certain components
|
||
// of the Software may be covered by so-called “open source” software licenses (“Open Source
|
||
// Components”), which means any software licenses approved as open source licenses by the
|
||
// Open Source Initiative or any substantially similar licenses, including without limitation any
|
||
// license that, as a condition of distribution of the software licensed under such license,
|
||
// requires that the distributor make the software available in source code format. Licensor shall
|
||
// provide a list of Open Source Components for a particular version of the Software upon
|
||
// Licensee’s request. Licensee will comply with the applicable terms of such licenses and to
|
||
// the extent required by the licenses covering Open Source Components, the terms of such
|
||
// licenses will apply in lieu of the terms of this Agreement. To the extent the terms of the
|
||
// licenses applicable to Open Source Components prohibit any of the restrictions in this
|
||
// License Agreement with respect to such Open Source Component, such restrictions will not
|
||
// apply to such Open Source Component. To the extent the terms of the licenses applicable to
|
||
// Open Source Components require Licensor to make an offer to provide source code or
|
||
// related information in connection with the Software, such offer is hereby made. Any request
|
||
// for source code or related information should be directed to cl-face-tracker-distribution@lists.cam.ac.uk
|
||
// Licensee acknowledges receipt of notices for the Open Source Components for the initial
|
||
// delivery of the Software.
|
||
|
||
// * Any publications arising from the use of this software, including but
|
||
// not limited to academic journal and conference publications, technical
|
||
// reports and manuals, must cite at least one of the following works:
|
||
//
|
||
// OpenFace: an open source facial behavior analysis toolkit
|
||
// Tadas Baltrušaitis, Peter Robinson, and Louis-Philippe Morency
|
||
// in IEEE Winter Conference on Applications of Computer Vision, 2016
|
||
//
|
||
// Rendering of Eyes for Eye-Shape Registration and Gaze Estimation
|
||
// Erroll Wood, Tadas Baltrušaitis, Xucong Zhang, Yusuke Sugano, Peter Robinson, and Andreas Bulling
|
||
// in IEEE International. Conference on Computer Vision (ICCV), 2015
|
||
//
|
||
// Cross-dataset learning and person-speci?c normalisation for automatic Action Unit detection
|
||
// Tadas Baltrušaitis, Marwa Mahmoud, and Peter Robinson
|
||
// in Facial Expression Recognition and Analysis Challenge,
|
||
// IEEE International Conference on Automatic Face and Gesture Recognition, 2015
|
||
//
|
||
// Constrained Local Neural Fields for robust facial landmark detection in the wild.
|
||
// Tadas Baltrušaitis, Peter Robinson, and Louis-Philippe Morency.
|
||
// in IEEE Int. Conference on Computer Vision Workshops, 300 Faces in-the-Wild Challenge, 2013.
|
||
//
|
||
///////////////////////////////////////////////////////////////////////////////
|
||
|
||
#include <Face_utils.h>
|
||
|
||
// OpenCV includes
|
||
#include <opencv2/core/core.hpp>
|
||
#include <opencv2/imgproc.hpp>
|
||
|
||
// For FHOG visualisation
|
||
#include <dlib/opencv.h>
|
||
|
||
using namespace std;
|
||
|
||
namespace FaceAnalysis
|
||
{
|
||
|
||
// Pick only the more stable/rigid points under changes of expression
|
||
void extract_rigid_points(cv::Mat_<double>& source_points, cv::Mat_<double>& destination_points)
|
||
{
|
||
if(source_points.rows == 68)
|
||
{
|
||
cv::Mat_<double> tmp_source = source_points.clone();
|
||
source_points = cv::Mat_<double>();
|
||
|
||
// Push back the rigid points (some face outline, eyes, and nose)
|
||
source_points.push_back(tmp_source.row(1));
|
||
source_points.push_back(tmp_source.row(2));
|
||
source_points.push_back(tmp_source.row(3));
|
||
source_points.push_back(tmp_source.row(4));
|
||
source_points.push_back(tmp_source.row(12));
|
||
source_points.push_back(tmp_source.row(13));
|
||
source_points.push_back(tmp_source.row(14));
|
||
source_points.push_back(tmp_source.row(15));
|
||
source_points.push_back(tmp_source.row(27));
|
||
source_points.push_back(tmp_source.row(28));
|
||
source_points.push_back(tmp_source.row(29));
|
||
source_points.push_back(tmp_source.row(31));
|
||
source_points.push_back(tmp_source.row(32));
|
||
source_points.push_back(tmp_source.row(33));
|
||
source_points.push_back(tmp_source.row(34));
|
||
source_points.push_back(tmp_source.row(35));
|
||
source_points.push_back(tmp_source.row(36));
|
||
source_points.push_back(tmp_source.row(39));
|
||
source_points.push_back(tmp_source.row(40));
|
||
source_points.push_back(tmp_source.row(41));
|
||
source_points.push_back(tmp_source.row(42));
|
||
source_points.push_back(tmp_source.row(45));
|
||
source_points.push_back(tmp_source.row(46));
|
||
source_points.push_back(tmp_source.row(47));
|
||
|
||
cv::Mat_<double> tmp_dest = destination_points.clone();
|
||
destination_points = cv::Mat_<double>();
|
||
|
||
// Push back the rigid points
|
||
destination_points.push_back(tmp_dest.row(1));
|
||
destination_points.push_back(tmp_dest.row(2));
|
||
destination_points.push_back(tmp_dest.row(3));
|
||
destination_points.push_back(tmp_dest.row(4));
|
||
destination_points.push_back(tmp_dest.row(12));
|
||
destination_points.push_back(tmp_dest.row(13));
|
||
destination_points.push_back(tmp_dest.row(14));
|
||
destination_points.push_back(tmp_dest.row(15));
|
||
destination_points.push_back(tmp_dest.row(27));
|
||
destination_points.push_back(tmp_dest.row(28));
|
||
destination_points.push_back(tmp_dest.row(29));
|
||
destination_points.push_back(tmp_dest.row(31));
|
||
destination_points.push_back(tmp_dest.row(32));
|
||
destination_points.push_back(tmp_dest.row(33));
|
||
destination_points.push_back(tmp_dest.row(34));
|
||
destination_points.push_back(tmp_dest.row(35));
|
||
destination_points.push_back(tmp_dest.row(36));
|
||
destination_points.push_back(tmp_dest.row(39));
|
||
destination_points.push_back(tmp_dest.row(40));
|
||
destination_points.push_back(tmp_dest.row(41));
|
||
destination_points.push_back(tmp_dest.row(42));
|
||
destination_points.push_back(tmp_dest.row(45));
|
||
destination_points.push_back(tmp_dest.row(46));
|
||
destination_points.push_back(tmp_dest.row(47));
|
||
}
|
||
}
|
||
|
||
// Aligning a face to a common reference frame
|
||
void AlignFace(cv::Mat& aligned_face, const cv::Mat& frame, const LandmarkDetector::CLNF& clnf_model, bool rigid, double sim_scale, int out_width, int out_height)
|
||
{
|
||
// Will warp to scaled mean shape
|
||
cv::Mat_<double> similarity_normalised_shape = clnf_model.pdm.mean_shape * sim_scale;
|
||
|
||
// Discard the z component
|
||
similarity_normalised_shape = similarity_normalised_shape(cv::Rect(0, 0, 1, 2*similarity_normalised_shape.rows/3)).clone();
|
||
|
||
cv::Mat_<double> source_landmarks = clnf_model.detected_landmarks.reshape(1, 2).t();
|
||
cv::Mat_<double> destination_landmarks = similarity_normalised_shape.reshape(1, 2).t();
|
||
|
||
// Aligning only the more rigid points
|
||
if(rigid)
|
||
{
|
||
extract_rigid_points(source_landmarks, destination_landmarks);
|
||
}
|
||
|
||
cv::Matx22d scale_rot_matrix = LandmarkDetector::AlignShapesWithScale(source_landmarks, destination_landmarks);
|
||
cv::Matx23d warp_matrix;
|
||
|
||
warp_matrix(0,0) = scale_rot_matrix(0,0);
|
||
warp_matrix(0,1) = scale_rot_matrix(0,1);
|
||
warp_matrix(1,0) = scale_rot_matrix(1,0);
|
||
warp_matrix(1,1) = scale_rot_matrix(1,1);
|
||
|
||
double tx = clnf_model.params_global[4];
|
||
double ty = clnf_model.params_global[5];
|
||
|
||
cv::Vec2d T(tx, ty);
|
||
T = scale_rot_matrix * T;
|
||
|
||
// Make sure centering is correct
|
||
warp_matrix(0,2) = -T(0) + out_width/2;
|
||
warp_matrix(1,2) = -T(1) + out_height/2;
|
||
|
||
cv::warpAffine(frame, aligned_face, warp_matrix, cv::Size(out_width, out_height), cv::INTER_LINEAR);
|
||
}
|
||
|
||
// Aligning a face to a common reference frame
|
||
void AlignFaceMask(cv::Mat& aligned_face, const cv::Mat& frame, const LandmarkDetector::CLNF& clnf_model, const cv::Mat_<int>& triangulation, bool rigid, double sim_scale, int out_width, int out_height)
|
||
{
|
||
// Will warp to scaled mean shape
|
||
cv::Mat_<double> similarity_normalised_shape = clnf_model.pdm.mean_shape * sim_scale;
|
||
|
||
// Discard the z component
|
||
similarity_normalised_shape = similarity_normalised_shape(cv::Rect(0, 0, 1, 2*similarity_normalised_shape.rows/3)).clone();
|
||
|
||
cv::Mat_<double> source_landmarks = clnf_model.detected_landmarks.reshape(1, 2).t();
|
||
cv::Mat_<double> destination_landmarks = similarity_normalised_shape.reshape(1, 2).t();
|
||
|
||
// Aligning only the more rigid points
|
||
if(rigid)
|
||
{
|
||
extract_rigid_points(source_landmarks, destination_landmarks);
|
||
}
|
||
|
||
cv::Matx22d scale_rot_matrix = LandmarkDetector::AlignShapesWithScale(source_landmarks, destination_landmarks);
|
||
cv::Matx23d warp_matrix;
|
||
|
||
warp_matrix(0,0) = scale_rot_matrix(0,0);
|
||
warp_matrix(0,1) = scale_rot_matrix(0,1);
|
||
warp_matrix(1,0) = scale_rot_matrix(1,0);
|
||
warp_matrix(1,1) = scale_rot_matrix(1,1);
|
||
|
||
double tx = clnf_model.params_global[4];
|
||
double ty = clnf_model.params_global[5];
|
||
|
||
cv::Vec2d T(tx, ty);
|
||
T = scale_rot_matrix * T;
|
||
|
||
// Make sure centering is correct
|
||
warp_matrix(0,2) = -T(0) + out_width/2;
|
||
warp_matrix(1,2) = -T(1) + out_height/2;
|
||
|
||
cv::warpAffine(frame, aligned_face, warp_matrix, cv::Size(out_width, out_height), cv::INTER_LINEAR);
|
||
|
||
// Move the destination landmarks there as well
|
||
cv::Matx22d warp_matrix_2d(warp_matrix(0,0), warp_matrix(0,1), warp_matrix(1,0), warp_matrix(1,1));
|
||
|
||
destination_landmarks = cv::Mat(clnf_model.detected_landmarks.reshape(1, 2).t()) * cv::Mat(warp_matrix_2d).t();
|
||
|
||
destination_landmarks.col(0) = destination_landmarks.col(0) + warp_matrix(0,2);
|
||
destination_landmarks.col(1) = destination_landmarks.col(1) + warp_matrix(1,2);
|
||
|
||
// Move the eyebrows up to include more of upper face
|
||
destination_landmarks.at<double>(0,1) -= 30;
|
||
destination_landmarks.at<double>(16,1) -= 30;
|
||
|
||
destination_landmarks.at<double>(17,1) -= 30;
|
||
destination_landmarks.at<double>(18,1) -= 30;
|
||
destination_landmarks.at<double>(19,1) -= 30;
|
||
destination_landmarks.at<double>(20,1) -= 30;
|
||
destination_landmarks.at<double>(21,1) -= 30;
|
||
destination_landmarks.at<double>(22,1) -= 30;
|
||
destination_landmarks.at<double>(23,1) -= 30;
|
||
destination_landmarks.at<double>(24,1) -= 30;
|
||
destination_landmarks.at<double>(25,1) -= 30;
|
||
destination_landmarks.at<double>(26,1) -= 30;
|
||
|
||
destination_landmarks = cv::Mat(destination_landmarks.t()).reshape(1, 1).t();
|
||
|
||
LandmarkDetector::PAW paw(destination_landmarks, triangulation, 0, 0, aligned_face.cols-1, aligned_face.rows-1);
|
||
|
||
// Mask each of the channels (a bit of a roundabout way, but OpenCV 3.1 in debug mode doesn't seem to be able to handle a more direct way using split and merge)
|
||
vector<cv::Mat> aligned_face_channels(aligned_face.channels());
|
||
|
||
for (int c = 0; c < aligned_face.channels(); ++c)
|
||
{
|
||
cv::extractChannel(aligned_face, aligned_face_channels[c], c);
|
||
}
|
||
|
||
for(size_t i = 0; i < aligned_face_channels.size(); ++i)
|
||
{
|
||
cv::multiply(aligned_face_channels[i], paw.pixel_mask, aligned_face_channels[i], 1.0, CV_8U);
|
||
}
|
||
|
||
if(aligned_face.channels() == 3)
|
||
{
|
||
cv::Mat planes[] = { aligned_face_channels[0], aligned_face_channels[1], aligned_face_channels[2] };
|
||
cv::merge(planes, 3, aligned_face);
|
||
}
|
||
else
|
||
{
|
||
aligned_face = aligned_face_channels[0];
|
||
}
|
||
}
|
||
|
||
|
||
void Visualise_FHOG(const cv::Mat_<double>& descriptor, int num_rows, int num_cols, cv::Mat& visualisation)
|
||
{
|
||
|
||
// First convert to dlib format
|
||
dlib::array2d<dlib::matrix<float,31,1> > hog(num_rows, num_cols);
|
||
|
||
cv::MatConstIterator_<double> descriptor_it = descriptor.begin();
|
||
for(int y = 0; y < num_cols; ++y)
|
||
{
|
||
for(int x = 0; x < num_rows; ++x)
|
||
{
|
||
for(unsigned int o = 0; o < 31; ++o)
|
||
{
|
||
hog[y][x](o) = *descriptor_it++;
|
||
}
|
||
}
|
||
}
|
||
|
||
// Draw the FHOG to OpenCV format
|
||
auto fhog_vis = dlib::draw_fhog(hog);
|
||
visualisation = dlib::toMat(fhog_vis).clone();
|
||
}
|
||
|
||
// Create a row vector Felzenszwalb HOG descriptor from a given image
|
||
void Extract_FHOG_descriptor(cv::Mat_<double>& descriptor, const cv::Mat& image, int& num_rows, int& num_cols, int cell_size)
|
||
{
|
||
|
||
dlib::array2d<dlib::matrix<float,31,1> > hog;
|
||
if(image.channels() == 1)
|
||
{
|
||
dlib::cv_image<uchar> dlib_warped_img(image);
|
||
dlib::extract_fhog_features(dlib_warped_img, hog, cell_size);
|
||
}
|
||
else
|
||
{
|
||
dlib::cv_image<dlib::bgr_pixel> dlib_warped_img(image);
|
||
dlib::extract_fhog_features(dlib_warped_img, hog, cell_size);
|
||
}
|
||
|
||
// Convert to a usable format
|
||
num_cols = hog.nc();
|
||
num_rows = hog.nr();
|
||
|
||
descriptor = cv::Mat_<double>(1, num_cols * num_rows * 31);
|
||
cv::MatIterator_<double> descriptor_it = descriptor.begin();
|
||
for(int y = 0; y < num_cols; ++y)
|
||
{
|
||
for(int x = 0; x < num_rows; ++x)
|
||
{
|
||
for(unsigned int o = 0; o < 31; ++o)
|
||
{
|
||
*descriptor_it++ = (double)hog[y][x](o);
|
||
}
|
||
}
|
||
}
|
||
}
|
||
|
||
// Extract summary statistics (mean, stdev, min, max) from each dimension of a descriptor, each row is a descriptor
|
||
void ExtractSummaryStatistics(const cv::Mat_<double>& descriptors, cv::Mat_<double>& sum_stats, bool use_mean, bool use_stdev, bool use_max_min)
|
||
{
|
||
// Using four summary statistics at the moment
|
||
// Means, stds, mins, maxs
|
||
int num_stats = 0;
|
||
|
||
if(use_mean)
|
||
num_stats++;
|
||
|
||
if(use_stdev)
|
||
num_stats++;
|
||
|
||
if(use_max_min)
|
||
num_stats++;
|
||
|
||
sum_stats = cv::Mat_<double>(1, descriptors.cols * num_stats, 0.0);
|
||
for(int i = 0; i < descriptors.cols; ++i)
|
||
{
|
||
cv::Scalar mean, stdev;
|
||
cv::meanStdDev(descriptors.col(i), mean, stdev);
|
||
|
||
int add = 0;
|
||
|
||
if(use_mean)
|
||
{
|
||
sum_stats.at<double>(0, i*num_stats + add) = mean[0];
|
||
add++;
|
||
}
|
||
|
||
if(use_stdev)
|
||
{
|
||
sum_stats.at<double>(0, i*num_stats + add) = stdev[0];
|
||
add++;
|
||
}
|
||
|
||
if(use_max_min)
|
||
{
|
||
double min, max;
|
||
cv::minMaxIdx(descriptors.col(i), &min, &max);
|
||
sum_stats.at<double>(0, i*num_stats + add) = max - min;
|
||
add++;
|
||
}
|
||
}
|
||
}
|
||
|
||
void AddDescriptor(cv::Mat_<double>& descriptors, cv::Mat_<double> new_descriptor, int curr_frame, int num_frames_to_keep)
|
||
{
|
||
if(descriptors.empty())
|
||
{
|
||
descriptors = cv::Mat_<double>(num_frames_to_keep, new_descriptor.cols, 0.0);
|
||
}
|
||
|
||
int row_to_change = curr_frame % num_frames_to_keep;
|
||
|
||
new_descriptor.copyTo(descriptors.row(row_to_change));
|
||
}
|
||
|
||
} |